How do You Test or Implement use Cases for AI
Artificial Intelligence (AI) has separated itself as a key component of modern advancement which disrupts entire industries and revolutionizes how business is conducted. But, the correct application of AI requires precise and well thought out testing and execution. This article focuses on the primary steps and factors that are necessary to consider when testing and implementing AI use cases.
Table of Contents
Understanding AI Use Cases
What Are AI Use Cases?
AI use cases are the particular instances or particular use cases where AI is used to solve a problem or provide additional value. These cases cut across multiple domains such as health, banking, retail, and other industries. For example, AI enabled chatbots in customer support or predictive analytics in supply chain management are perfect examples of AI use cases in different business functions.
Why Is It Important to Test AI Use Cases?
AI enables algorithms to work with large data fundaments but still misinterpret the information fed into them, resulting in biased or erroneous outcomes. Thus testing AI use cases becomes critical so that AI systems are able to deliver on their promises. Trust for AI becomes difficult without precision testing, which can lead to operational failure. Hence the use of comprehensive testing helps validate models and systems during the design process.
Step-by-Step Guide to Implementing AI Use Cases
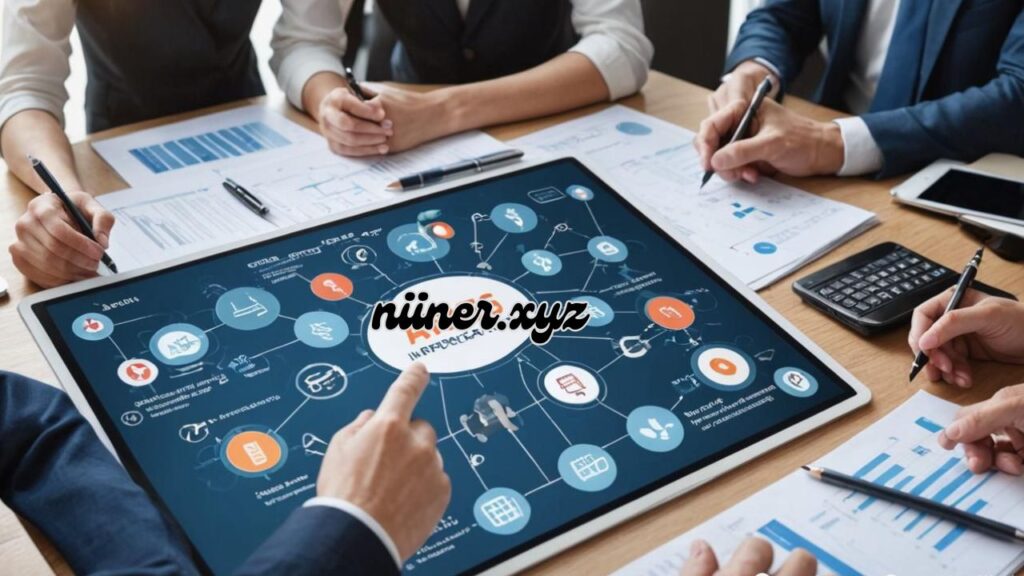
1. Identifying Business Needs and AI Opportunities
The process starts by identifying where AI can have maximum impact. This requires examining the current processes and pinpointing pain points or problems that can be solved with AI technologies. AI outreach activities that resonate with business objectives can yield the most value for an organization. This is accomplished through strategic alignment.
2. Defining the AI Use Case
Clearly, stating the problem to be addressed is imperative after identifying possible opportunities. This encompasses defining goals and setting key performance indicators (KPIs) for benefit realization. Use cases that are well formulated facilitate development and set expectations for stakeholders.
3. Data Collection and Preparation
There is no denying that data is an essential ingredient for AI. Therefore, The first and most importantes step is gathering relevant data of high quality. This stage includes cleaning, tagging and preprocessing data, which guarantees that they appropriately reflect the problem space in question. Good data preparation is key to preemptive AI modeling.
4. Choosing the Right AI Model and Algorithms
One of the critical aspects is choosing the right AI model. In reference to the problem, a person may choose supervised, unsupervised, or reinforcement learning algorithms. The context of the use case together with the type of data provided should dictate the choice of model to be used.
5. Developing and Training the AI Model
Having communicated the Data and algorithms, the next steps involves building and training the model, A process that require achieving a careful balance between performance and generalization, overseeing overfitting and underfitting restrictions. Model accuracy is improved by iteratively testing and validating its performance.
Testing AI Use Cases for Accuracy and Reliability
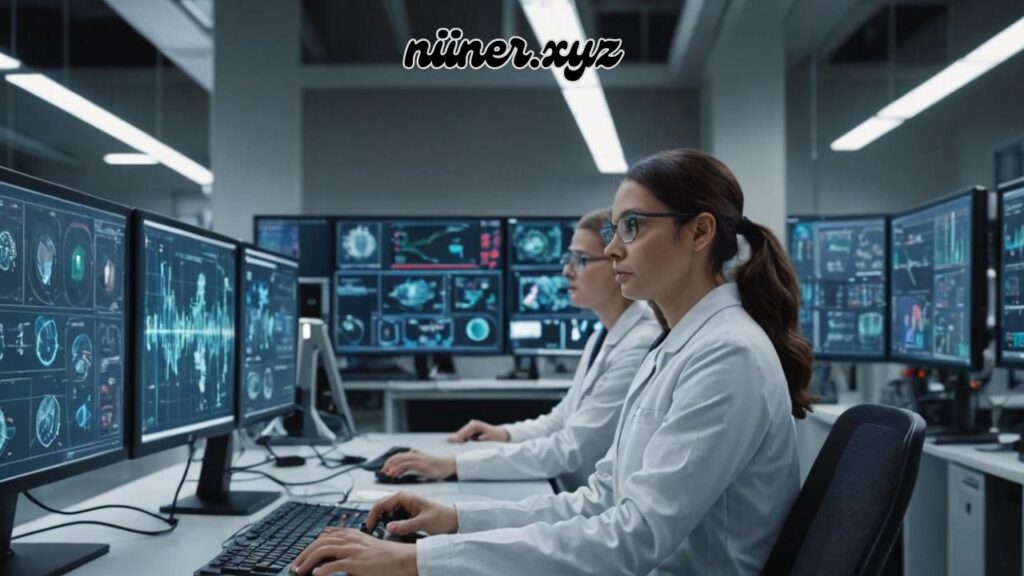
6. Creating a Testing Framework
It is important to have a structured testing framework as this is crucial for any system evaluation AI models. Such a framework’s structure must cover success criteria and outline the scope of the model’s performance assessment.
7. Performing Model Validation and Evaluation
Some techniques such as cross validation help determine how the AI model performs on unseen data. Other key performance evaluation metrics like precision, recall, or even F1 measure provide useful insights into the performance of the model as well as its shortcomings.
8. Running Real-World Testing and Simulations
No full blown deployment should be made before adequate real world testing has been conducted, through pilot programs and simulations. This ensures the organization gets to see the AI system working in real scenarios, as well as pinpoints possible issues and correcting them.
Challenges in Testing and Implementing AI Use Cases
9. Common AI Testing Challenges
Some of the unique issues in AI systems testing include but are not limited to:
- Non-deterministic Outputs: Output to inputs provided to AI models is not deterministic because of the probabilistic nature of AI systems.
- Data Quality Issues: Biased data especially if inaccurate will provide an equally erroneous model.
- Integration Issues: Integrating AI systems with the other existing systems of the organization eases the intercommunication but poses a challenge in co-existing for the different components.
10. Ethical and Legal Considerations
Adoption of AI comes with ethical and legal obligations. Issues such as data privacy, discrimination, and biased decision making require full attention to transparency and responsible AI.
Deployment and Continuous Monitoring of AI Models
11. Deploying AI in Production Environments
Moving AI models from the development phase into production calls for thorough strategizing. To incorporate real-world systems, efforts should be made towards scalability, reliability, and performance optimization to meet business objectives.
12. Monitoring and Improving AI Performance Over Time
Post-deployment, it is always important to actively monitor for things such as model drift and other performance degrading factors. Frequent changes made to the system also aid in keeping the AI system fresh and accurate to current requirements.
Future Trends in AI Use Case Implementation
13. The Role of Explainable AI (XAI)
As AI systems change, so does the importance of being able to describe their decisions. Reasonable AI is intended at creating AI systems that are responsible and assist in overseeing the system instead than allowing it act as a black box.
14. Emerging Technologies in AI Testing and Implementation
New breakthroughs in AI are grabbing attention all around the world. Automatic testing frameworks and AI-oriented development tools are accelerating the process of implementing AI, leading to AI adoption across the board.
Conclusion
Achieving success while testing AI use cases is not simple nor singular as it comprises of multiple pieces that need to be put together taking into account planning, ethics, and vigilance. Open systems and structures along with holistic perspective enable organizations to leverage the promising aspects of AI responsibly.
FAQs
- What piece of information is the single most vital when putting an AI use case through a test?
- The quality and the relevance of the data is the most important factor because it affects the effectiveness of the AI model directly.
- In what ways do you make sure fairness and lack of bias persist within AI models?
- Reducing biases in AI tools can be achieved by carrying out frequent audits, utilizing various data sets, and Including fairness limitations during model training.
- What solutions can you suggest for AI testing and monitoring?
- In the market, there are many users who are familiar with Applitools for visual testing, or Testim for automated functional testing.
- What can small companies do to begin adopting AI use cases?
- Small companies should start with identifying clear problems where AI can be utilized and look for appropriate problem solving whether it is scalable or suitable to the company’s resources.
- What risks do badly tested AI systems pose?
- Risks such as inaccurate predictions, biased results, and even ethical and legal issues are all possible outcomes that may occur from second-rate AI systems.